A new pattern recognition method for abnormal event detection in crowded scenarios
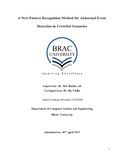
View/ Open
Date
4/18/2017Publisher
BRAC UniversityAuthor
Mostafa, Tahjid AshfaqueMetadata
Show full item recordAbstract
We propose an autonomous video surveillance system which analyzes surveillance footages of extremely crowded scenes and detects abnormal events. For any particular scenario, any event that diverts from the usual pattern can be classified as an abnormal event. The model analyzes the local spatial-temporal motion pattern and detects abnormal motion variations and sudden changes. It can be divided into two major parts, selecting a set of Points of Interest (POI) from given frames using ORB (Oriented FAST and Rotated BRIEF) feature detector and tracking them across multiple frames and dividing the input video frame in a number of cubes and track the motion patterns in each of the cubes for spatial-temporal statistical deviations. To evaluate the performance of proposed model we utilize several datasets and compare the acquired results of the proposed model with various state-of-the art models. Experimental results demonstrate that the proposed model outperforms the other models by exhibiting an average of 96.12% accuracy using Convolutional Neural Network.