Skin lesion classification using different CNN models
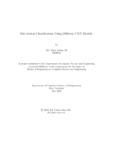
View/ Open
Date
2023-05Publisher
Brac UniversityAuthor
Oli, Md. Yahea SultanMetadata
Show full item recordAbstract
The field of dermatoscopic image classification has gained significant attention as
there is a growing demand for early diagnosis of specific diseases. The use of deep
learning is increasingly significant in the quest for a more effective dermoscopic anal-
ysis method. The “HAM10000” (Human Against Machine) dataset has been used in
this study for classification of 7 different types of skin lesions by using DenseNet-121,
VGG16, ResNet50, and Inceptionv3 model. To improve the classifier’s performance,
data augmentation was applied. This study could help dermatologists in the clinic
make more precise decisions when identifying skin lesions, which would be benefi-
cial. With this project I have tried to improve the model so that dermatologists
identify skin lesions more precisely. Through the implementation of data augmen-
tation techniques, this project achieved an impressive categorical accuracy of 92%
and a top2 accuracy of 97% using DenseNet-121. The remaining models, VGG16,
ResNet50, Inceptionv3 achieved accuracy 80%, 78%, 84% respectively. This project
could have a beneficial impact on dermatoscopic image recognition and can reduce
time and valuable resources. It can also help to saves life where robust diagnosing
is not available.