LRFS: online shoppers’ behavior based efficient customer segmentation model
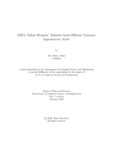
View/ Open
Date
2023-02Publisher
Brac UniversityAuthor
Khan, Riyo HayatMetadata
Show full item recordAbstract
The popularity of online shopping has grown significantly across the globe in recent
years. This research proposes a customer segmentation model LRFS, an extended
version of LRF model, built specifically for online shopping, using a dataset that
includes some features taken from Google Analytics. It introduces component S,
which measures the Staying Rate across the Revenue spent by the customers on
a particular website, to get a better insight into the customer base. Three wellknown clustering methods K-Means, K-Medoids, and DBSCAN algorithms were
incorporated along with the proposed model. For each of these algorithms, the
dataset was compressed separately using three different dimensionality techniques
such as PCA, t-SNE, and Autoencoder to figure out the combinations that could
work well for the used dataset. A comparative analysis has also been conducted
among LR, LF, LRF, and the proposed LRFS model using K-Means clustering.
LRFS has outperformed the other three models in terms of better cluster assignment
of the customers. For customer analysis, a combined Customer Classification and
Customer Relationship Matrix was used to determine the clustered groups according
to their characteristics. The combination of K-Median and t-SNE was chosen for
the final combined matrix since it had the highest number of most distinct clusters
with all traits of customer groups. Finally, some test cases as well as related use
case scenarios have been described and visualized using LRFS along with K-Means
and PCA.