Classification of Bangla regional languages and recognition of artificial Bangla speech using deep learning
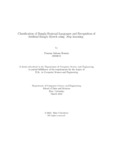
View/Open
Date
2022-03Publisher
Brac UniversityAuthor
Hossain, Prommy SultanaMetadata
Show full item recordAbstract
Since 1970, researchers have been attempting to recognize and comprehend spontaneous
speech. For an automatic voice recognition system, many techniques were
employed. People always choose English for voice recognition since it has been the
subject of the majority of study and implementation. However, Bangla is fifth most
widely spoken languages in the world. Bangla regional language voice recognition
has the potential to have a significant influence on human-computer interaction and
internet of things applications. Majority of the research performed in the past decade
in Bangla speech recognition involves classification of age, gender, speaker identification
and detection of specific words. However, classification of regional Bangla
language from Bangla speech and the identification of artificial Bangla speech has
not been researched heavily before. Due to the limitation of grammatical and phonetic
database with various Bangla regional language. Hence the author of this paper
has created 30 hours of Bangla regional language speech dataset, that covers the
dialect spoken by the locals in seven districts/division of Bangladesh. Bangla speech
was generated, by first converting Bangla words to English word aberration (used
often as text language) that would ultimately translate to a English phrase. Additionally,
to classify the regional language spoken by the speaker in the audio signal
and determine its authenticity, the suggested technique was used. Stacked convolutional
autoencoder (SCAE) and sequence of multi-label extreme learning machines
(MLELMs). SCAE section of the model creates a detailed feature map from Mel
Frequency Energy Coefficients (MFECs) input data by identifying the spatial and
temporal salient qualities. Feature vector is then fed to the first MLELM network to
produce soft classification score for each data. Based on which the second MLELM
network would generate hard labels. The suggested method was excessively trained
and tested on unsupervised data which is the formation of new sentence from the
unique Bangla/English abbreviation words. The model is also able to categorization
speaker’s characteristic such as; age and gender. Through experimentation it was
found that the model generates better accuracy score label for regional language
with taking age class into consideration. As aging generates physiological changes
in the brain that alter the processing of aural information, increasing classification
accuracy from 75% to 92% without and with age class consideration, respectively.
This was able to be achieved due to the usage of MLELMs networks, input data is a
multi labeled dataset, that classify labels based on linked patterns between classes.
The classification accuracy for synthesised Bangla speech labels 93%, age 95%, and
gender class label 92%. The proposed methodology works well with English speech
audio-set as well.