Real-time fire detection based on feature analysis using enhanced color segmentation and novel foreground extraction
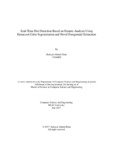
View/ Open
Date
2017-07Publisher
Brac UniversityAuthor
Khan, Rubayat AhmedMetadata
Show full item recordAbstract
This research proposes two effective real time fire detection techniques, based on video processing. The former technique is restricted to indoor conditions only while the later does not have such constraints. Both the proposed methods utilize prominent features such as flame color information and spatiotemporal characteristics to identify fire areas. For the first technique, color segmentation is carried out in the earliest stage to separate potential fire areas using the red component of RGB. Moving pixels are identified using frame differences from a reference frame followed by de-noising. In the next phase of the model, the growth of the segmented regions of the current frame is compared with later frames and based on the fact that a hazardous fire expands with time, regions with no or decreasing growth is removed. The complex boundary of fire (rotundity) is valuable information that aids in detection. In the last step, a feature vector is created with rotundity information and trained using a neural network. The proposed model is tested using a dataset containing a wide range of indoor lighting conditions and compared to a state of the art fire detection technique to confirm its effectiveness. The experimental results show that the proposed model performs better compared to the state of the art model in terms of accurate detection and computation time, yielding an average accuracy of 99.1%.For the second technique, the initial stage of the work extracts fire colored pixels using a set of enhanced rules on RGB. Fire pixels are dynamic and to detect these moving pixels a novel method is proposed in this approach. The final verification is done by examining the area of the extracted regions. A harmful fire will grow over time, thus if the area happens to increase, the region under focus is declared as fire. Experimental results show that the model put forward outperforms other state of art models yielding an accuracy of 97.7%.