Effective feature selection for real-time stock trading in variable time-frames and multi criteria decision theory based Efficient stock portfolio management
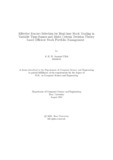
View/ Open
Date
2021-08Publisher
Brac UniversityAuthor
Ullah, A. K. M. AmanatMetadata
Show full item recordAbstract
The unpredictability and volatility of the stock market render it challenging to
make a substantial pro t using any generalized scheme. Many previous studies tried
di erent techniques to build a machine learning model, which can make a signi cant
pro t in the US stock market by performing live trading. However, very few studies
have focused on the importance of nding the best features for a particular period for
trading. Our top approach used the performance to narrow down the features from a
total of 148 to about 30. Furthermore, the top 25 features were dynamically selected
before each time training our machine learning model. It uses ensemble learning with
four classi ers: Gaussian Naive Bayes, Decision Tree, Logistic Regression with L1
regularization and Stochastic Gradient Descent, to decide whether to go long or short
on a particular stock. Our best model performed daily trade between July 2011 and
January 2019, generating 54.35% pro t. We further propose a novel model which
uses Ada-boost to nd the weights of each of the features and then apply TOPSIS
to select the best stocks. Lastly, we survey the machine learning techniques used
for ethical decision-making in stock trading, which will bene t any further research
work on Responsible AI in Finance.