Emotion detection using EEG signals
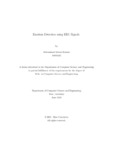
View/ Open
Date
2021-06Publisher
Brac UniversityAuthor
Hossain, Mohammad AdnanMetadata
Show full item recordAbstract
Emotions play a vital role in how people feel, think and act which makes it worthwhile for analyzing human behavior. As the patterns of emotions and their reflections differ from person to person, their study needs to be based on methods that are
effective regardless of the diverse domain of the population. Hence, the analysis of
physiological signals in detecting and extracting human emotions is gaining significance. To support this, resources and standards are being developed simultaneously.
In this paper, we propose a pre-processing method along with some feature extractions and a model for emotion detection using EEG Signals based on DEAP dataset,
a current benchmark for Emotion Classification research. For the pre-processing of
data, prominent channels which contribute most to the classification are selected
based on the role of the prefrontal cortex in emotion regulation and conscious experience and as for feature extractions, wavelet energy, wavelet entropy, and standard
deviation are used. DNN (Deep Neural Network), SVM (Support Vector Machine),
and KNN (K-Nearest Neighbour) are considered as the proposed model to detect
emotions on a quadrant, HAHV (High Arousal and High Valence) or HALV (High
Arousal and Low Valence) or LAHV (Low Arousal and High Valence) or LALV (Low
Arousal and Low Valence). The approach we used yielded a maximum accuracy of
64%, 64%, and 70% for valence, arousal, and dominance respectively.